Papyrus
http://hdl.handle.net/1866/23782
Abstract
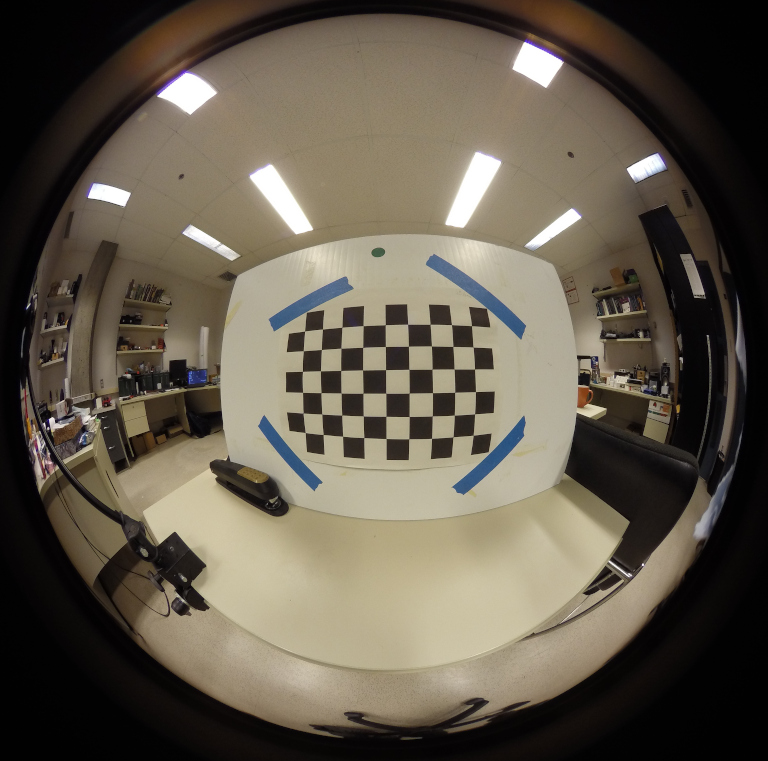
This thesis focuses on the problems of calibrating wide-angle cameras and estimating depth from a single camera, stationary or in motion. The work carried out is at the intersection between traditional 3D vision and new deep learning methods in the field of autonomous navigation. They are designed to allow the detection of obstacles by a moving drone equipped with a single camera with a very wide field of view. First, a new calibration method is proposed for fisheye cameras with very large field of view by planar calibration with dense correspondences obtained by structured light that can be modelled by a set of central virtual generic cameras. We demonstrate that this approach allows direct modeling of axial cameras, and validate it on synthetic and real data. Then, a method is proposed to estimate the depth from a single image, using only the strong depth cues, the T-junctions. We demonstrate that deep learning methods are likely to learn from the biases of their data sets and have weaknesses to invariance. Finally, we propose a method to estimate the depth from a camera in free 6 DoF motion. This involves calibrating the fisheye camera on the drone, visual odometry and depth resolution. The proposed methods allow the detection of obstacles for a drone.
Documents